AI adoption stays top-of-mind for companies. Although business are eager to acquire competitive benefit by leveraging AI to more quickly bring developments to market, they are typically not able to see outcome as rapidly as they ‘d like.
Troubles dealt with when moving designs into production consist of expense and an absence of automation– mentioned by over 55% of participants to a current IDC research study. 1 The intricacy of structure knowledge, handling numerous tools and platforms throughout the ML pipeline, and remaining on top of an ever-expanding repository of production designs are kept in mind as more challenges.
In a difficult economy, dexterity, speed, and performance are important. Business require reputable AI forecasts that fulfill company objectives so they can make educated choices and rapidly react to alter. This is why companies are significantly buying artificial intelligence operations (MLOps): IDC anticipates by 2024, 60% of business will have operationalized their ML workflows by utilizing MLOps. 2
What Is MLOps and How Does It Assist?
MLOps integrates individuals, procedures, finest practices and innovations that automate the implementation, tracking and management of ML designs into production. Through embracing MLOps practices and tools, companies can dramatically alter how they approach the whole ML lifecycle and provide concrete advantages.
The advantages of embracing MLOps tools and procedures consist of:
- Faster time to worth, and more quick function roll-out, through much better preparation and implementation practices;
- Much better run the risk of mitigation for production designs through continuous tracking, governance, and revitalize for underperforming designs;
- Faster shipment through enhanced cooperation for multi-functional groups generally associated with the ML lifecycle, such as information researchers, information engineers, and IT;
- Scalable AI techniques that can support lots and even numerous production designs.
Should You Construct or Purchase an MLOps Platform?
There are essential factors to consider when checking out MLOps. Understand how your company deals with ML– and where it needs to head. Recognize requirements concerning structure, releasing, tracking, and governing your ML designs on a holistic basis.
IDC suggests dealing with designs as source code to enhance cooperation, design reuse, and tracking. Ask even more concerns to assist your company strategy to enhance performance and dexterity when dealing with ML designs. How would it deal with scale and handling extra designs? How can you finest prevent replicating effort when handling ML designs throughout departments with various requirements, and provide more worth?
Dealing with a supplier will be advantageous. Utilize a cost-benefit analysis to check out ROI and danger. Not doing anything or moving too gradually might quickly and adversely affect your company. By contrast, injecting rate into your ML efforts can future-proof your company and keep it ahead of the competitors.
You’ll discover chances and expense compromises– and clear benefits in buying an MLOps option. These might consist of:
- more quickly producing company returns
- much better leveraging knowings
- decreased requirement for specialized workers
- flexible reasonings for expense management
- automated scale throughout your company
- effective design operations from a main management system
How Is DataRobot MLOps Distinctively Placed to Handle ML Difficulties?
When you deal with a recognized and relied on software application supplier, it is very important to select one that will conserve you money and time, and assist you effectively and efficiently handle the numerous obstacles that included developing AI jobs or speeding up AI adoption. With DataRobot MLOps, you get a center of quality for your production AI– a single location to release, handle and govern designs in production, despite how they were developed or when and where they were released.
This complete suite of ML lifecycle abilities provides design screening, implementation and serving, efficiency tracking and granular model-level insights, approval workflows, and a greater level of self-confidence for choices notified by designs. Information science groups can then much better attend to obstacles related to the ML lifecycle.
Although it’s loaded with functions, DataRobot MLOps is likewise simple to utilize. Amongst its numerous highlights are:
- A single pane of glass management console that combines reporting, with quickly absorbable charts, workflow evaluation, and quality metrics;
- Custom-made AI task governance policies, providing you total control over gain access to, evaluation, and approval workflows throughout your company;
- Automating much of the ML advancement procedure, consisting of tracking, production diagnostics, and implementation, to enhance the efficiency of existing designs;
- Running your designs anywhere, through DataRobot MLOps having the ability to release your design to a production environment of option;
- The market leading DataRobot AutoML, which develops and evaluates opposition designs– and signals you and supplies insights when one outshines the champ;
- A humbleness function, which sets up guidelines to make it possible for designs that acknowledge in real-time when they ensure forecasts;
- Comprehensive and user-defined insights, which let you, for instance, compare drift throughout 2 scoring sections of a design, for whenever duration, to acquire the context needed to effectively make crucial choices that keep designs pertinent in a fast-changing world.
MLOps is a need to stay competitive in today’s difficult financial environment. DataRobot MLOps assists you more quickly make the most of the great chances ML brings, and effectively and efficiently handle the lifecycle of production designs holistically throughout your whole business.
For a much deeper dive into the topics of this post, consisting of more assistance on the MLOps area, and to see why DataRobot was called a Leader, have a look at the “ IDC MarketScape: Worldwide Artificial Intelligence Operations Platforms 2022” report. You’ll likewise find more about how DataRobot MLOps can assist your business handle ML obstacles.
1 Source: “IDC MarketScape: Worldwide Artificial Intelligence Operations Platforms 2022 Supplier Evaluation,” doc #US 48325822, December 2022
2 Source: “IDC FutureScape: Worldwide Expert System and Automation 2022 Forecasts,” IDC #US 48298421, October 2021
About the author
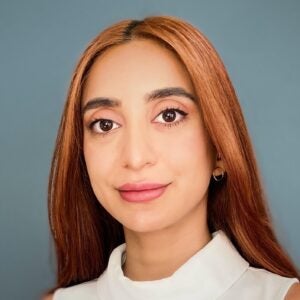
Data Researcher, DataRobot
Might Masoud is an information researcher, AI supporter, and believed leader trained in classical Stats and modern-day Artificial intelligence. At DataRobot she develops market technique for the DataRobot AI Cloud platform, assisting international companies obtain quantifiable return on AI financial investments while keeping business governance and principles.
Might established her technical structure through degrees in Stats and Economics, followed by a Master of Company Analytics from the Schulich School of Company. This mixed drink of technical and company knowledge has actually formed Might as an AI professional and an idea leader. May provides Ethical AI and Equalizing AI keynotes and workshops for company and scholastic neighborhoods.