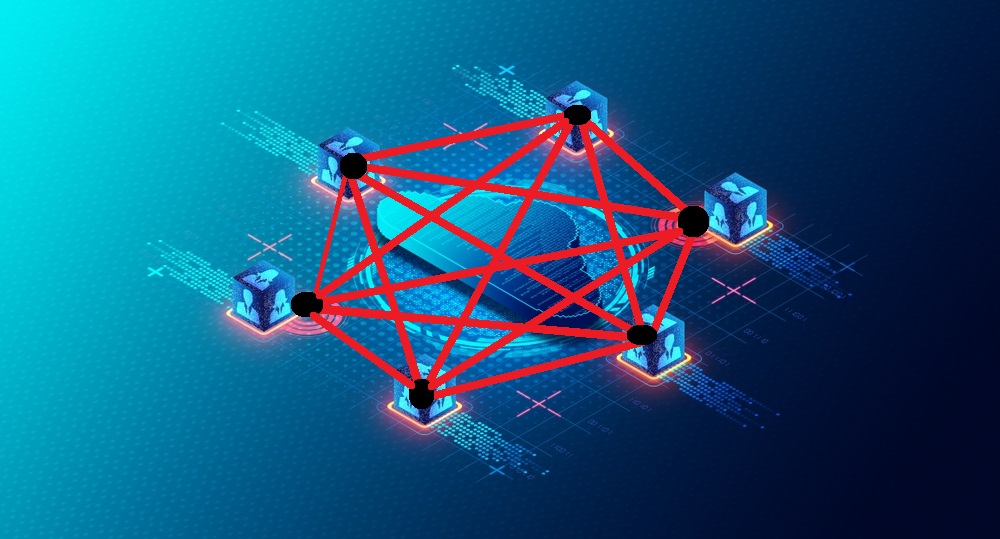
(ArtemisDiana/Shutterstock)
Kobai this week introduced the release of Saturn, a brand new providing designed to carry the facility of the data graph to information already saved in lakehouses. By way of making a semantic layer that sits atop Snowflake and Databricks lakehouses, consumers achieve the aptitude to run SPARQL queries towards that information, giving them a formidable new strategy to glean insights however with out the complexity of a full-blown graph database venture.
Kobai was once based 5 years in the past by means of two former instrument engineers at Basic Electrical, Ryan Oattes and Parag Goradia. The pair struggled to lend a hand GEâs business consumers construct information methods that would monitor and question the state of itemsâsimilar to all of the components in an planeâover a longer time period.
âWe had been in reality curious about the type of complexity that comes when you wish to have 10 mavens to unravel the in reality arduous issues, such because the lifestyles of a few section that was once designed, manufactured, serviced over 25 years,â says Oattes, Kobaiâs CTO. âThere are such a large amount of other people you wish to have to have collaborate round âHow can we construction information?â and âWhat are the analytics we wish to do on it?â When youâre handing it off to builders, that simply makes it more difficult.â
An RDF-based wisdom graphâwith a triple-store constructed round a topic, object, and predicateâwas once a really perfect strategy to prepare this type of data. However as a substitute of beginning on the database stage and increase, Oattes and Goradia opted to start on the different finish: the consumer interface. Their first providing, Kobai Studio, had the end-user entrance and middle.
âWe donât get started speaking about information in our engagements. We commence with industry customers, mavens who say âLetâs speak about your first 5 or 10 questions. What are the items of your online business that you wish to have to learn about?ââ Oattes tells Datanami. âOne of the most first workers was once a UX fashion designer. How was once a consumer going to engage with this? How can we make it like two or 3 guys participating across the whiteboard that then turns into actionable?â
Maximum Kobai implementations leveraged Virtuoso, an open supply graph database evolved by means of OpenLink Device (even if anything else with a SPARQL endpoint will paintings). Whilst Virtuoso has served many Kobai shoppers neatly, itâs in keeping with Postgres and subsequently has restricted scalability. That has confirmed to be a dealbreaker for larger deployments by means of Kobaiâs greater consumers.
So in overdue 2021, the parents at Kobai began growing Saturn. The function with Saturn necessarily is to construct a data graph that leverages the dimensions that Databricks and Snowflake have already evolved of their lakehouse choices.
Saturn mainly purposes as a virtualization layer between Kobai Studio and the underlying lakehouse from Snowflake or Databricks. This sediment takes the SPARQL queries generated by means of Kobai Studio and interprets it into SQL code, which Snowflake and Databricks expect. Saturn then returns the question consequence to the Kobai Studio consumer, the place it may energy a dashboard or be utilized in in a different way.
âWe’ve got some IP round how can we construction a relational schema and deal with it over the years in Databricks or Snowflake this is amenable to performant graph queries being run throughout it,â Oattes says. Â âSo we’re consuming the knowledge right into a schema in Databricks or Snowflake this is arranged by means of Kobai. Itâs the Saturn schema inside Databricks or Snowflake, after which from there, while youâre operating queries towards it, youâre operating them via form of a Kobai provider on best.â
Kobai was once interested in Snowflake and Databricks for a number of causes. The obvious is that the 2 lakehouse suppliers are attracting a large amount of information. 1000’s of shoppers are parking their information in those two lakehouses, and making an investment sources to regulate their information there. By way of enforcing a data graph provider layer atop the lakehouse, Kobai can leverage the ones present buyer investments.
âWe’re writing queries which can be optimized for Photon within the Databricks case,â Oattes says, including that theyâve carried out the similar for Snowflake. âItâs tuned to these environments. So we get to journey on best of that funding that Databricks and Snowflake have made when it comes to the funding they’ve made.â
The lakehouses additionally supplies lower price of possession for Kobai consumers, in addition to workload isolation, which turns into a subject matter with greater implementations. If a Kobai Studio consumer kicks off an in depth graph question that can contact the similar piece of information that Snowflake or Databricks is querying to energy a dashboard, the Kobai consumer doesnât have to fret about efficiency problems that may consequence when the ones workloads collide.
âWith a lakehosue, we want one replica of the knowledge and I will be able to isolate the compute for either one of the ones use circumstances,â Oattes says.
By way of pairing Kobai Studio with Saturn within the cloud, the Pleasanton, California corporate now has the dimensions to satisfy a few of its larger consumersâ graph wishes. The corporateâs greatest consumer is a Fortune 100 aviation conglomerate with the prior to now discussed parts-tracking problem. Itâs additionally running with consumers that experience computational demanding situations in monetary products and services and team of workers control, Oattes says.
One in every of Kobaiâs consumers needs to optimize their provide chain operations now not round value, however round chance, similar to environmental, social, and governance (ESG) variables.
âWhat if I wish to herald now not simply geopolitical chance, however ESG stuff?â Oattes says. âIf I would like in an effort to make choices in keeping with â¦.the human rights report of a few of these providers? Numerous conventional BI retail outlets are roughly unprepared for the versatility required to carry that stuff in.â
Along with bringing scale and workload isolation to Kobaiâs wisdom graph consumers, it additionally let them enlarge their wisdom graph out to new parts in their industry that havenât but benefited from this era. That has the possible to snare extra information into the RDF scheme of items, yielding better advantages because it grows, Oattes says.
âAs a substitute of the usage of an example of Kobai for a staff or a ventureâ¦this shall we me embody the community impact of the knowledge material, the place each and every use case extends this logical ontology throughout my industry,â Oattes says. âAnd now I am getting further worth from having that broader context to be had for me, coaching fashions, dashboardsâno matter it’s.â
Kobai is beginning with Snowflake and Databricks with Saturn, however the corporate has plans to enlarge to different lakehouses sooner or later. For more information, see the corporateâs website online at www.kobai.io.
Similar Pieces:
Knowledge Materials: The Killer Use Case for Wisdom Graphs
Databricks Claims 30x Merit within the Lakehouse, However Does It Hang Water?
Why Younger Builders Donât Get Wisdom Graphs
Â